pandas基础使用
挑选列
按照boolean index(即逻辑表达式)
Pandas Select DataFrame columns using boolean
comb.loc[:, criteria1 & criteria2]
如挑选含有\N
的列:
tag.loc[:, tag.isin(['\\N']).any()]
对应的挑选不含\N
的列:
tag.loc[:, (~tag.isin(['\\N'])).all()]
丢弃元素(drop)
整一行丢弃
关键在于挑选index:
df = df.drop(df[<some boolean condition>].index)
df.drop(df[<some boolean condition>].index, inplace=True)
不使用科学记数法
全局
pd.set_option('display.float_format',lambda x : '%.2f' % x)
局部(针对某一列)
参考 Finer Control: Display Values
简单处理
这种方法只能一次性的,因为style只是返回一个Style
对象,而不是一个Dataframe
df.style.format({'B': "{:0<4.0f}", 'D': '{:+.2f}'})
正确的做法应该如下:
TypeError: ‘Styler’ object is not subscriptable
# apply style on the columns
df.style.apply(lambda x: ["text-align:right"]*len(x))
或者直接转化为string
self_analysis['group_id'] = self_analysis['group_id'].apply(lambda x:'%.0f' % x)
设置显示相关一些选项(最大宽度,最大行树,列数等等)
import pandas as pd
pd.set_option('display.max_rows', 500)
pd.set_option('display.max_columns', 500)
pd.set_option('display.width', 1000)
按index访问
Python Pandas: Get index of rows which column matches certain value
需要注意:
- index必须是有序的(即如果前面有用排序sort_values,需要重新reset_index)
- 例子
df.index[df['BoolCol'] == True].tolist()
查看某个值是否在Dataframe/Series中
how to check if a value exists in a dataframe
直接用isin()
,会返回一个Boolean的Dataframe或者Series
groupby的使用
agg函数
- 传入的func所接收的参数默认只是一个Series!
apply函数
- 传入func所接收的参数是分组后的整个dataframe
List of tuples转化为Dataframe
最佳方法:直接转换为dict,然后最外面再套层dict即可
Remap values in pandas column with a dict
>>> df = pd.DataFrame({'col2': {0: 'a', 1: 2, 2: np.nan}, 'col1': {0: 'w', 1: 1, 2: 2}})
>>> di = {1: "A", 2: "B"}
>>> df
col1 col2
0 w a
1 1 2
2 2 NaN
>>> df.replace({"col1": di})
col1 col2
0 w a
1 A 2
2 B NaN
append行到Dataframe
Is it possible to append Series to rows of DataFrame without making a list first?
主要思路:
- 转化为Series
- 然后直接append,且设定
ignore_index=True
对于MultiIndex的问题,可以先把索引保存到一个变量里面,append,然后对MultiIndex做修改,最后再重新设定index
# 一开始不设index
cur_res_df = pd.DataFrame(cur_res,columns=['num_insts', 'auc', 'loss', 'rig', 'l_avg', 'p_avg', 'uauc'])
cur_get_total_avg = partial(get_total_avg, num_vec=cur_res_df['num_insts'])
# 转化为Series或者直接获得Series
total_avg = cur_res_df.iloc[:, 1:].apply(cur_get_total_avg, axis=0).append(pd.Series({'num_insts': '/'}))
cur_res_df = cur_res_df.append(total_avg, ignore_index=True)
# 设定MultiIndex
iterables = [[model_name], dt_hr_idx + [dt_hr_idx[0].split('/')[0]+'_avg']]
multi_idx = pd.MultiIndex.from_product(iterables)
cur_res_df.index = multi_idx
MultiIndex根据level获得index具体值
具体的值,tuple类型
df.index.to_list()
直接获得一个list of dict
tt.index.to_list()
Out[6]:
[('0620/12', '0610_ckpt_on_0620'),
('0620/12', '0617_ckpt_on_0620'),
('0620/13', '0610_ckpt_on_0620'),
('0620/13', '0617_ckpt_on_0620'),
('0620/14', '0610_ckpt_on_0620'),
('0620/14', '0617_ckpt_on_0620'),
('0620/15', '0610_ckpt_on_0620'),
('0620/15', '0617_ckpt_on_0620'),
('0620/16', '0610_ckpt_on_0620'),
('0620/16', '0617_ckpt_on_0620'),
('0620/17', '0610_ckpt_on_0620'),
('0620/17', '0617_ckpt_on_0620'),
('0620/18', '0610_ckpt_on_0620'),
('0620/18', '0617_ckpt_on_0620'),
('0620/19', '0610_ckpt_on_0620'),
('0620/19', '0617_ckpt_on_0620'),
('0620_avg', '0610_ckpt_on_0620'),
('0620_avg', '0617_ckpt_on_0620')]
获得各个层级具体的值(带重)
df.index.get_level_values(0)
根据需要更改层级即可
tt.index.get_level_values(0)
Out[11]:
Index([u'0620/12', u'0620/12', u'0620/13', u'0620/13', u'0620/14', u'0620/14', u'0620/15', u'0620/15', u'0620/16', u'0620/16', u'0620/17',
u'0620/17', u'0620/18', u'0620/18', u'0620/19', u'0620/19', u'0620_avg', u'0620_avg'],
dtype='object')
获得各个层级具体的值(去重)
df.index.levels[0]
tt.index.levels[0]
Out[12]: Index([u'0620/12', u'0620/13', u'0620/14', u'0620/15', u'0620/16', u'0620/17', u'0620/18', u'0620/19', u'0620_avg'], dtype='object')
用MultiIndex选择元素
level 0+具体某一列
直接.loc[level0_index, colum_name]
tt.loc['0620/12', 'num_insts']
Out[18]:
0610_ckpt_on_0620 1239040
0617_ckpt_on_0620 1239040
选择level=1 + 某一列/某几列
最方便最直接就是用 pandas.IndexSlice,这也是官方教程中所推荐的:Using slicers
使用pandas.IndexSlice后,能够像numpy的slicer那样方便
例子:
选择0617_ckpt的所有列:
idx = pd.IndexSlice
all_shuffled_slots_df.loc[idx[:, '0617_ckpt'], idx[:]]
Out[13]:
auc l_avg loss num_insts p_avg rig uauc
0619_avg_on_shuffled_shuffled_246 0617_ckpt 0.55606 0.23897 2.45143 20000 0.32099 -3.46041 0.55606
0619_avg_on_shuffled_shuffled_2 0617_ckpt 0.49614 0.23897 4.27246 20000 0.41853 -6.77546 0.49614
选择0617_ckpt和auc,uauc列:
all_shuffled_slots_df.loc[idx[:, '0617_ckpt'], idx['auc', 'uauc']]
Out[14]:
auc uauc
0619_avg_on_shuffled_shuffled_246 0617_ckpt 0.55606 0.55606
0619_avg_on_shuffled_shuffled_2 0617_ckpt 0.49614 0.49614
MultiIndex丢弃某个或某些level的索引
可以用于把一些共用的,没有含义的索引丢弃 How to remove levels from a multi-indexed dataframe?
all_shuffled_slots_df.loc[idx[:, model['disp']], idx[:]]
Out[36]:
auc l_avg loss num_insts p_avg rig uauc
0619_avg_on_shuffled_shuffled_246 all_0617-0617_non_pretrain 0.55608 0.23897 2.45143 20000 0.32099 -3.46041 0.55608
0619_avg_on_shuffled_shuffled_2 all_0617-0617_non_pretrain 0.49604 0.23897 4.27246 20000 0.41853 -6.77546 0.49604
all_shuffled_slots_df.loc[idx[:, model['disp']], idx[:]].reset_index(level=1, drop=True)
Out[37]:
auc l_avg loss num_insts p_avg rig uauc
0619_avg_on_shuffled_shuffled_246 0.55608 0.23897 2.45143 20000 0.32099 -3.46041 0.55608
0619_avg_on_shuffled_shuffled_2 0.49604 0.23897 4.27246 20000 0.41853 -6.77546 0.49604
尝试更改整个Dataframe的Columns:ValueError: Length mismatch: Expected axis has 2 elements, new values have 3 elements
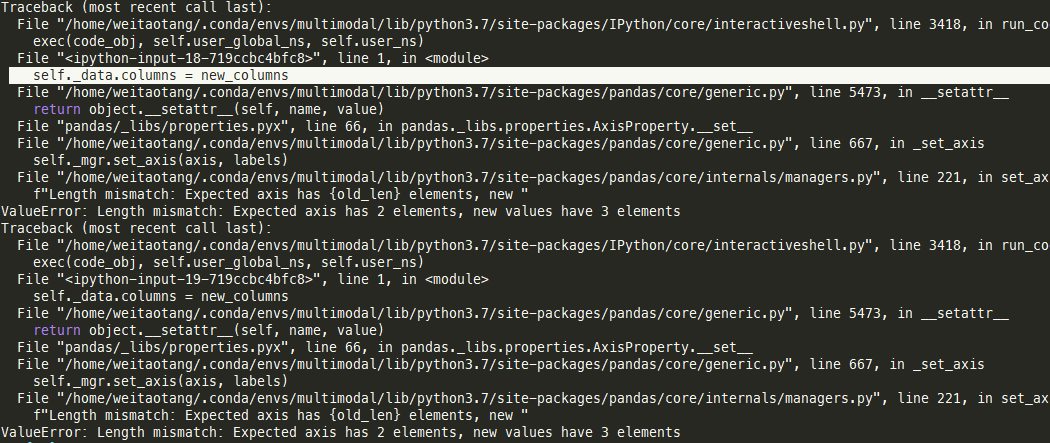
原因:
The problem is that you have an empty data frame which has zero columns, and you are trying to assign a four columns multi-index to it; If you create an empty data frame of four columns initially, the error will be gone:
即Dataframe的Columns大小一旦创建后就是固定的,如果axis的elements数对不上,是没办法更改的(本质上MultiIndex可以看做很多单个elements组成)。这样的设定也很好理解:一旦Dataframe中有数据之后,保证数据不会丢失
解决方法:
- 一开始初始化时就初始化好固定的index
- or直接创建一个新的Dataframe